목록전체 글 (110)
David의 개발 이야기!
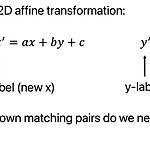
A. Least Squares Suppose we need an affine transformation to align one image to the other one. 위 식을 정리를 하면 아래처럼 표현할 수 있다. 정리된 식을 미분하면, 세타 에 대한 식이 나오는데, 여기 양변에 A 를 곱해주면, A * theta = b 식이 나오게 된다. 따라서, 해당 문제를 푸는것이 문제가 된다. 즉, image alignment 를 위해서, A, B에서 interest point 를 찾고, 이를 이으면서, homography 를 추정한다. homography 행렬은, 한 이미지의 관심점들을 다른 이미지의 관심점으로 변환하는데 사용할 수 있으며, 두 이미지간의 변환을 구현할 수 있다. B. RANSAC : Ran..
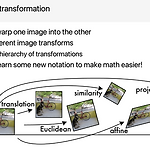
A. Geometric Transformation 1. Geometric Transformation 예시 2. Homogeneous Coordinate 설명 위의 homogeneous coordinate 개념에 따라, 아래와 같이 homogrphy matrix 를 만들수 있다. B. Image warping 이미지를 저렇게 회전시키려면 어떻게 해야할까? Forward warping 이라고 하는데, 수식을 통해, rotate gkrh, scaling을 변환한다. 하지만, 이럴 경우, 아래 이미지처럼 비어있는 값이 생기는 문제가 발생한다. 그래서 근본적인 문제를 해결하기 위해 "backward mapping"을 사용한다. forward mapping과 backward mapping의 차이는 아래와 같다. t..
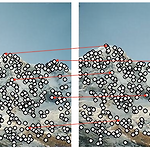
시작하기 앞서, 용어를 설명하려고 한다. Feature Description 은, 여러 필터와 detector 들을 통해 검출된 feature points 주변의 정보를 요약하여, 그 특징점을 기술하는 단게이다. 이 과정은, 특징점의 위치뿐만 아니라, 그 특징점이 어떤 외형적인 특성을 가지고 있는지 포착하는 것을 목표로 한다. 이는, 다른 이미지에서 같은 특징점을 식별하고 매칭하는데 사용할 수 있는 정보를 제공한다. 우리는 이전 포스팅까지, 어떻게 interest points들을 찾는지 배웠다. 이제 우리의 다음 목표는, corresponding pair를 찾는것이다! 위의 작업은 매우 어려운 작업중 하나인데, 왜냐하면, 이미지의 색깔도 고려해야하며, 또, 다양한 appearance 및 various g..
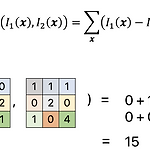
1. Harris Corner Detector (Cont) 자 이전 포스팅까지, edge와 corner를 찾는 방법에 대해 배웠다. 우리는 지금 파노라마를 만드는 방법을 위한 기반을 공부하고 있는데, 어떻게 하면, 두 지점의 유사성을 판단할 수 있을까? 라는 질문을 던져보아야한다. 방법은 아래 이미지와 같다. 유사성을 판단하는 방법을 알게 되었으니, 그 다음으로 고민해야할 것은, "어떻게 독특한 고유의 patch를 찾을 것인가?" 이다. 모든 지점을 일일히 위와 같은 방식으로 계산하게 된다면, 엄청난 Computing Loss가 날 것이다. patch가 너무 많기 때문이다 그렇기 때문에, 우리는, 보통 local neighbor 만 고려하는 방식을 채택한다. 좋은 feature란, 자신을 유일하게 특정지..
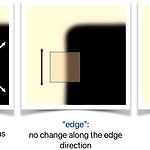
본 포스팅에서는 파노라마를 만드는 방법에 대해 기술 하고자 한다. 파노라마를 배우는 이유는, CV에서, 초급, 중급에 해당하는 기초적인 알고리즘이기 때문이다. 파노라마를 만드는 방식은 아래 순서와 같다. 1. Detect Interest Points 2. Describe small patches about such points 3. Find Matches between pairs of such points on two images 4. Find geometric transformations from one image to the other. 5. Warp one image to the other to align them 6. Blend the image together. 1. How can we find..
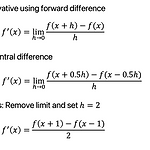
A. Image Gradient 1. How to detect Image Edges? Q1 ) How would you go about detecting edges in an image? => Take derivatives: derivatives are large at discontinuities. 미분은 이미지에서 강도가 급격히 변하는 영역을 강조한다. 이러한 영역은 대개 엣지에 해당한다. Q2) How do you differentiate a discrete image (or any other discrete signal)? => Use finite differences 디지털 이미지는 연속적이지 않고, 이산적이다. 이미지는 픽셀로 구성되며, 각 픽셀은 이산적인 강도 값을 가진다. 이는 연속함수에 사..